A Canadian scientist has studied the issue of the causes of global warming and resultant climate change, from an entirely different direction.
Shaun Lovejoy (PhD) is a professor of Physics at McGill University in Montreal, and president of non-linear processes section at the European Geosciences Union.
ListenMost scientific studies related to global warming demonstrate and show man’s affect on the climate. Nevertheless, no modeling is perfect and claims of “natural fluctuation” persist and are promoted by skeptics who continually claim the models are wrong, inaccurate or incomplete.
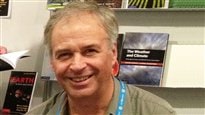
Professor Lovejoy took the “Sherlock Holmes” approach in that if you eliminate the alternatives, you are left only with the probable answer.
The study published this month in the online journal Climate Dynamics, is called ” Scaling fluctuation analysis and statistical hypothesis testing of anthropogenic warming”
“This study will be a blow to any remaining climate-change deniers,” S. Lovejoy (PhD)
What professor Lovejoy did was therefore to study the competing hypothesis of natural causes or variation to explain warming of the earth since the start of the industrial age.
![Thisfigure visually shows the strong linear relation between the radiative forcing and the global temperature responsesince1880, it is a simplified version of fig.3a of [Lovejoy, 2014a] showing the 5 year running average of global temperature (red) as a function of the CO2forcing surrogate from 1880 to 2004. The linearity is impressive; the deviations from linearity are due to natural variability. The slope of the regression line is 2.33 ± 0.22 oC per CO2 doubling (it is for the unlagged forcing/response relation). CLICK to ENLARGE](https://www.rcinet.ca/en/wp-content/uploads/sites/3/2014/04/LOVEJOY-WARM-GRAPH-240x135.jpg)
This figure visually shows the strong linear relation between the radiative forcing and the global temperature response since 1880, it is a simplified version of fig.3a of [Lovejoy, 2014a] showing the 5 year running average of global temperature (red) as a function of the CO2forcing surrogate from 1880 to 2004.
The linearity is impressive; the deviations from linearity are due to natural variability. The slope of the regression line is 2.33 ± 0.22 oC per CO2 doubling (it is for the unlagged forcing/response relation).
CLICK to ENLARGE
All of this combined gave a picture of “natural” temperature and climate fluctuations over ranges of time scales.
By testing the natural fluctuation hypothesis, he was able to show clearly that the recent rise of global temperature since the beginning of the industrial age, or approximately 125 years, was not natural at all. Basically the current increase in global temperature in this short a time lapse, has probably not occurred in the last several thousand years and so is extremely unlikely to be due to any “natural” cause
“Natural causes may be ruled out with 99% certainty” Lovejoy (PhD)
In fact, while coming at the issue from the opposite direction, in terms of CO2 levels and temperature rise, he was able to get results which are very similar to the many computer models from the vast number of climate studies and figures in the report of the Intergovernmental Panel on Climate Change (IPCC)
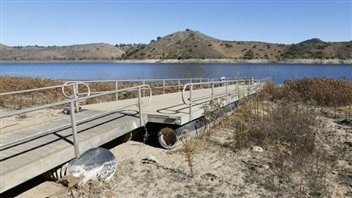
“This study will be a blow to any remaining climate-change deniers,” Lovejoy says. “Their two most convincing arguments – that the warming is natural in origin, and that the computer models are wrong – are either directly contradicted by this analysis, or simply do not apply to it.”
His study, using the relationship between global economic activity and the emission of greenhouse gases also included the cooling effect of particulate pollution which he says are generally not well quantified in most climate models.
His analysis shows that the amount of warming, about 0.9 degrees Celsius in the historically brief time since 1880, is a huge and abnormal increase in such a very short time.
He says the odds of that occurring naturally are likely less than one in a thousand and the natural-warming hypothesis may be ruled out “with confidence levels great than 99%, and most likely greater than 99.9%.”
Professor Lovejoy notes that ruling out one hypothesis doesn’t necessarily prove the other, but often as in this case, it lends increased credibility to that alternative of man-made global warming
**** LOVEJOY RESPONSE TO QUESTIONS /CRITICS
(17.4.14)
Global temperature variations, fluctuations,
errors and probabilities Questions and Answers about the Climate Dynamics paper
I have been flooded with questions and purported rebuttals of my paper and
cannot answer them all individually. Here are some common misconceptions,
misunderstandings and in some cases misrepresentations of my paper. A (legal not
copyright protected) pre-proof version of the paper [Lovejoy, 2014a] can be found
here:
http://www.physics.mcgill.ca/~gang/eprints/eprintLovejoy/neweprint/Anth
ro.climate.dynamics.13.3.14.pdf.
Q. It is impossible to determine climate sensitivity either to the claimed precision
of 0.01 Cº or to 99% confidence from the temperature data.
A. This is a misrepresentation: I never claimed that I could estimate the
climate sensitivity to that accuracy – my value was 3.08±0.58 oC i.e. 95% of the time
between 3.08-2×0.58 and 3.08+2×0.58 equivalently between 1.9 and 4.2 oC for a CO2
doubling, and the confidence itself was not simply 99% but in a range of 99% to
99.99% with 99.9% the most likely.
Q. OK, so what about the ±0.03oC global annual surface temperature error
estimate just after eq. 1 in the paper?
A. The short answer is that the issue of accuracy of the surface measurements
is overblown, misunderstood and – unless it is far larger than any estimates give – it
is nearly irrelevant to the final conclusion (the number ±0.03 oC is from a paper a
year old, [Lovejoy et al., 2013],
http://www.physics.mcgill.ca/~gang/eprints/eprintLovejoy/neweprint/ESDD.com
ment.14.1.13.pdf).
If you really want to know the details, see the section for the statistically
inclined (below). The following Q and A also gives more details.
Q. Why did you ignore the temperature measurement errors?
A. Because the key error is the estimate of the anthropogenic component of
the warming – which was estimated as ±0.11 oC. This estimate is mostly due to the
uncertainty in the correct lag between the forcing and the response (zero to 20
years). The uncertainty (±0.11 oC) is so much larger than ±0.03 oC that the latter is
irrelevant.
2
Q. I think eq. 1 is a con. You simply define the natural variability as the residue
of a regression with the CO2 forcing, you can’t separate out the anthropogenic and
natural components in this way.
A. Equation 1 is motivated by the fact that anthropogenic effects – what ever
they are – don’t affect the statistical type of variability, essentially they affect the
atmospheric boundary conditions, so that the equation is at least plausible. But
you’re right that a priori it could be totally wrong. However the reason that we can
be confident that it isn’t wrong is because the residues have nearly the same
probability distributions and generally same statistics as the pre-industrial
multiproxies (see the Q and A below). Therefore the hypothesis that they really are
natural is verified (just check with your eye fig. 5, or do it properly, fig. 8).
Q. Your claim to be a nonlinear geophysicist makes me laugh. Your eq. 1 and
hypothesis about the CO2 RF surrogate is totally linear, you’re a hypocrite!
A. You’re the one missing a subtlety. I never said that in Equation 1, that Tnat
would have been the same with or without the anthropogenic changes/forcings.
That would indeed be a linearity assumption. I only hypothesize that the statistical
type of variability is the same.
This means that if I had two identical planets and on one of them, we let
humans loose while on the other, there were none, then the Tnat would be totally
different in their details but that their statistics (such as their standard deviations)
at all scales would be the same. In other words, eq. 1 does not contradict the fact
that there can (and will) be nonlinear interactions between the anthropogenic
forcings and the “internal” atmospheric dynamics.
Q. But the IPCC estimates the anthropogenic warming as 0.85 ± 0.20 oC (0.65 to
1.05 to oC) which has much larger range, larger uncertainty – how does that affect the
results?
A. The research for the paper was done before last September when the AR5
came out. I therefore used the AR4 (2007) range of 0.74±0.18 oC i.e. 0.56 to 0.92 oC.
The AR4 lower limit (0.56 oC) is a bit smaller than the AR5 lower limit (0.65 oC), so
that the more recent AR5 estimate makes us even more confident that the warming
is not natural. Anybody can use fig. 10 of the paper to check that with the AR5
values, the probability of the warming being natural goes down from 0.3 – 0.7%
(AR4) to 0.1 – 0.3% (depending on how extreme we assume the tails to be). My
lower limit 0.76 oC) gives a range of 0.05-0.2%.
Q. How big would the error have to be in order to make it plausible that the
industrial epoch warming is a just a natural fluctuation?
A. It depends on where your probability cut-off is. Let’s say that we’re ready
to accept that the warming was natural even if the probability was as low as 10%
(some people would reject the hypothesis at this level, but let’s see what it implies). 3
Using fig. 10, we find that this implies a change of only 0.26 oC. Therefore, even if
the temperature in 2013 was only 0.26 oC higher than it was 125 years ago, this
would still be surprising since it would only happen 10% of the time, but it might
nevertheless be a credible natural fluctuation.
Notice that the estimate 0.26 oC refers to an event that occurs over the entire
125 year period starting at its beginning, and whose probability of occurring is 10%
or less. This means that if we took 1250 years of the pre-industrial temperature
record and broke into ten 125 year segments, that only one of them would have a
change of 0.26 oC (or more) over its duration, all the others would have smaller
changes.
The actual value of the industrial period warming would therefore have to be
about three times smaller than current estimates in order for the natural warming
hypothesis to have any credibility.
Q. But the post-war cooling event was larger than 0.26 oC, surely there is a
mistake?
A. No, it is correct. The post-war cooling (1944-1976) turns out to be in the
range 0.42 – 0.47 oC (this includes an anthropogenic warming of 0.16 -0.21 oC, the
observed cooling is actually 0.26 oC; these estimates use the same methodology as
the Climate Dynamics paper and have been submitted to review elsewhere). But
according to fig. 10, such a large event is expected to occur every 100 years or so
(the “return period”). This can be also seen from the direct use of the 32 year curve
in fig. 9). However this is the biggest 32 year event we would expect in any 125
year period of time – no matter when it occurs within the 125 years – not starting at a
specific (given) point in time, here in 1880.
Thanks to natural variability, some event – with an amplitude equal to the post
war cooling – is thus expected to occur every century or so. Ex post facto, we know
that this event actually started in 1944. However, in 1880 we could only know that
such an event would almost surely occur at some point during the next 125 years.
Q. I still don’t understand how we can expect some 32 year event to be as big as
0.42 -0.47 oC yet we don’t expect the 125 year temperature difference from 1880 –
2004 to be nearly so big (less than 0.26 oC). How is this possible?
A. The reason is that from about 10 days until about 125 years, temperature
fluctuations tend to cancel each other out. This is the “macroweather regime” with
the property that over a time period Δt, the fluctuations in temperature ΔT tend to
decrease in a power law way: ΔT (Δt) ≈ ΔTH with H ≈ -0.1 for global temperatures.
([Lovejoy, 2013],
http://onlinelibrary.wiley.com/doi/10.1002/2013EO010001/pdf). Therefore if
there is a large random/natural excursion of temperature up, it tends to be followed
by one down that nearly cancels (and visa versa). For example, this is indeed the
case of the post-war cooling. It is also the case of the recent so-called “pause” – but
that’s another story for another paper! 4
Q. This has to wrong. If fluctuations always tend to cancel, then there would be
no medieval warming, no Little Ice Age and – for that matter – no Big Ice Age!
A. No, it correct. The reason is that the macroweather regime ends at about
100 – 125 year time scales and that the longer time scales have a lot more low
frequencies; the exponent H changes from -0.1 to about +0.4 so that and on the
contrary, the temperature fluctuations tend to grow with increasing time interval,
they tend to “wander”; this the true “climate” regime.
But of course this slow “wandering” doesn’t effect the shorter time scale 125
year fluctuations that are important here.
Q. I still don’t trust the global temperature series, what can I do?
A. The global series I picked are generally agreed to be the best available,
although since the analysis was done, they have had updates/improvements.
If you still don’t like them pick you own, then estimate the total change over a
125 year period and then use fig. 10 to work out the probability. You will find that
the probability rapidly decreases for changes larger than about 0.4oC – so as
discussed above – to avoid my conclusion, you’ll need to argue that the change in
temperature over the last 125 years is about a half to a third of what all the
instrumental data, proxy data and models indicate.
Q. But what about the Medieval warming with vines growing in Britain – or the
Little Ice Age and skating on the Thames? Surely the industrial epoch warming is just
another large amplitude natural event?
A. Well no. My result concerns the probability of centennial scale temperature
changes: large changes – if they occur slowly enough – are not excluded. So if you
must, let the peons roast and the Thames freeze solid, the result stands.
The question indicates a misunderstanding about the role of time resolution/
time scale. Taking differences at 125 years essentially filters out the low
frequencies.
Q. To estimate the probabilities you used the hockey stick and everyone knows
that it has been discredited, why should I believe your probabilities?
A. The hockey stick is the result of using a large number of paleo data to
reconstruct past global scale (usually annual resolution) temperatures. Starting
with the [Mann et al., 1998] series, there are now a dozen or so in the literature.
They have been criticized and improved, but not discredited. For example,
responding to early criticism, those published since 2003 have stronger low
frequency variability, thus rectifying some of the earlier limitations. But in spite of
some low frequency disagreements, as I have shown, even the pre and post 2003
multiproxies agree well with each other up to about 100-200 year scales which is all
that I need here (see fig. 9, 10 of [Lovejoy and Schertzer, 2012a], 5
http://www.physics.mcgill.ca/~gang/eprints/eprintLovejoy/neweprint/AGU.mono
graph.2011GM001087-SH-Lovejoy.pdf, and see also ch. 11 in [Lovejoy and
Schertzer, 2013].
Thus, while it is true that the multiproxies disagree somewhat with each other
at longer scales, this is irrelevant, only the statistics at 125 year scales are important
and up to this time scale they agree quite well with each other. As mentioned
before, it’s therefore possible that several (even all!) have underestimated the
medieval warming, but this is irrelevant to my conclusions.
Q. Why did you pick the multiproxies that you did: wouldn’t you get different
results with a different choice?
A. I picked a diverse group – one of the three (Huang) used boreholes (it
needed no paleo calibrations), one used a wavelet based method that helped to
ensure the low frequencies were realistic (Moberg), and one was an update of the
original Mann series with various improvements.
But as indicated, for the probability distribution of the differences of 125 years
and less, there isn’t much difference between any of them (note thatin principle the
series could be totally different but still have identical probabilities). Readers can
check the distributions in fig. 6 which show that the probabilities of differences are
nearly identical – except for a small difference in the fluctuation amplitudes. Such
differences are exactly what we expect if the amount of spatial averaging is a little
different in each (due to different mix of paleodata).
Q. How can you estimate a probability of an extreme (one in a thousand) event
that lasts a century? There isn’t anywhere near enough data for that!
A. This is the subject of the key second part of my study that uses the
multiproxy data from 1500 to estimate the probability that this temperature change
is due to natural causes. Since we are interested in rare, extreme fluctuations, a
direct estimate would indeed require far more pre-industrial measurements than
are currently available. This type of problem is standard in statistics and is usually
solved by applying the bell-curve. Using this, the chance of the fluctuation being
natural would be in the range of one in a hundred thousand to one in ten million. Yet
we know that climate fluctuations are much more extreme than those allowed by
the bell curve. This is where nonlinear geophysics comes in.
Nonlinear geophysics confirms that the extremes should be far stronger than
the usual “bell curve” allows. Indeed, I was able to show that giant century long
fluctuations are more than 100 times more likely than the bell curve would predict,
yet – at one in a thousand – their probability is still small enough that they can be
confidently rejected.
Q. You’re trying to con me with vague but sophisticated sounding gobbly-gook.
Give me more details, I’m still not convinced.
6
A. One needs to make an assumption about the probability tails (extremes). I
explicitly describe that assumption: that the distribution is asymptotically bounded
by power laws – and I give a theoretical justification from nonlinear geophysics:
power law probability tails (“fat tails”, associated with “black swan” huge
fluctuations) are generic features of scaling processes (such as the global
temperature up to about 125 years). Scaling also comes in because I use it to
extrapolate from the 64 year distributions to the 125 year distributions, but there is
big literature on this scaling (some of it is cited). Finally there is specific evidence
going back to 1985 (cited in the paper) for power law probabilities in climatological
temperatures including with the exponent qD = 5 (see [Lovejoy and Schertzer,
1986],
http://www.physics.mcgill.ca/~gang/eprints/eprintLovejoy/neweprint/Annales.G
eophys.all.pdf). Classical statistics assume Gaussian distributions (qD = infinity) and
the latter hypothesis would make the extremes 100 to 10000 even less likely. For
my 99% confidence result to be invalid, the power law would have to be incredibly
strong and start at probabilities below around 0.001. Even if you don’t like power
law tails – you might find them distastefully extreme and would feel more
comfortable with familiar Gaussians here they are only used as bounds. With
Gaussians, the actual probabilities would thus much smaller (as indicated above).
Q. What about cycles? Lots of people have claimed that temperatures vary
cyclically, couldn’t a cycle of the right length explain the warming?
A. No. The reason is that almost all of the variability (technically, the variance) is
in the “background” (continuum) part of the spectrum which as mentioned above, is
scaling (power law). The cycles may exist, but their contribution to the variability
(including overall changes) is tiny (with of course the exception of the annual cycle!).
See: [Lovejoy, 2014b],
http://www.physics.mcgill.ca/~gang/eprints/eprintLovejoy/esubmissions/climate.not.clim
ate.dynamics.7.2.14.pdf.
Q. It’s obvious that it’s the sun that causing the temperature to change, think about
the sunspots!
A. Solar forcings and responses are already taken care of in my analysis since Tnat
was defined to including anything not anthropogenic. However, at climate scales (longer
than 125 years), they might be important (e.g. for roasting the peons in 13th century
Britain). However, this is not trivial since according to sunspot based solar
reconstructions, we would need to find a mechanism that could nonlinearly amplify the
fluctuations by a factor 15 – 20, and this factor must apply over a wide range of time
scales (see [Lovejoy and Schertzer, 2012b],
http://www.physics.mcgill.ca/~gang/eprints/eprintLovejoy/neweprint/Sensitivity
.2012GL051871.proofs.SL.pdf). If we use the competing 10Be based reconstructions,
then the situation is hopeless since these reconstructions imply that rather than growing
with scale (H ≈ 0.4 as for the temperatures and sunspot based forcings), the
10Be based
reconstructions rapidly decrease with scale with H ≈-0.4. 7
Q. OK, forget the sun, it’s got to be volcanoes! We know that when they go off
there’s a huge effect think of Pinatubo or Krakatoa! Haven’t the last hundred years been
a bit calmer? (less volcanism, less volcanic cooling?).
A. The short answer is no, my analysis implicitly includes volcanism.
To make this more plausible, the beginning of my paper addresses this specifically
and shows that the last century of volcanism was a little weaker, but it was no big deal.
More to the point, when a volcano blows it’s a strong effect, but it doesn’t last long. Over
a century, you can forget it. According to fig. 1 (and see [Lovejoy and Schertzer,
2012b]), for volcanic forcing, H ≈ -0.4 so effects from volcanism (i.e. the time series
of volcanic forcings) rapidly cancel each other out.
Q. How can you ever prove that the warming was anthropogenic? As a scientist
you know that you have to do an experiment to prove anything, and we can’t rerun the
last 125 years without fossil fuels or humans just to see what would have happened.
You’ll never con me into believing that it’s our fault!
A. This is absolutely correct, but it is not what I claim!
I am not trying to prove that anthropogenic warming is correct, I’m trying to
disprove that natural warming is correct! Of course disproving natural warming makes
anthropogenic warming a lot more plausible, but it isn’t “proof” in a mathematical sense.
Maybe you’ll come up with some other alternative for example, a one-off miracle warmig
type event would not contradict my analysis (of course it would likely contradict the laws
of physics, but if you are to ready to suspend these…).
As I point out in the last sentence of my paper: “While students of statistics know
that the statistical rejection of a hypothesis cannot be used to conclude the truth of any
specific alternative, nevertheless – in many cases including this one – the rejection of one
greatly enhances the credibility of the other.“ (This last part is because we’re doing
science, not statistics!).
For the statistically minded, more error analysis
In equation 1 in my paper I mention that the average global temperatures can
be estimated to an accuracy of ±0.03 oC, and I refer to the source (fig. 1 bottom
curve, [Lovejoy et al., 2013]
http://www.physics.mcgill.ca/~gang/eprints/eprintLovejoy/neweprint/ESDD.com
ment.14.1.13.pdf). What does the figure show? I simply took four globally, annually
averaged surface temperature series from 1880 (NOAA, NASA, HAdcrut3 and the
Twentieth Century Reanalysis (20CR) series, [Compo et al., 2011]). These series
are all different, they use different data sets (although with overlap) and different
methodologies. In particular, one of them (the 20CR series) used no station
temperatures whatsoever, [Compo et al., 2013] (only station pressure data and
monthly averaged Sea Surface Temperatures are used) so for this one, there are no
issues of urban warming or stations being moved around, or tweaked or even 8
suffering from slow changes in day time –night time variability! They all agree with
each other to ±0.03K up to ≈ 100 year scales. (This number was estimated from the
root mean square of these differences which from the figure cited above is close to
0.05oC; if the corresponding variance is equally apportioned between the series, this
leads to about ±0.03 oC each. Finally, we’re interested in the differences at century
scales, and this allows us to do even more averaging, potentially doing even better,
see below for more details. Any biases from manipulation of temperature station
data must be small.
The basic reason that the errors are so small is that we are averaging
enormous numbers of measurements; if we take a large enough number we may
expect errors to cancel out and become negligible. For example, using standard
statistical assumptions about the independence of the errors, one expects that the
overall error decreases as the square root of the number of measurements.
As explained above, the one year differences have errors of about ±0.03oC, but
what about the systematic errors? How to quantify this?
I used a more sophisticated method of estimating the fluctuations between
each series and the mean of the four series. Its called Haar fluctuations (see:
[Lovejoy and Schertzer, 2012c]
http://www.physics.mcgill.ca/~gang/eprints/eprintLovejoy/Haar.npg-19-
513-2012.final.pdf). Over a time interval Δt, it is defined as the average of the first
half minus the average of the second half of the interval (i.e. for a century scale, this
would be the difference of the average of the first 50 years minus the average of the
next fifty years). Due to the averaging, this is quite robust, but also, since we can
change Δt, we can examine how quickly the errors decrease with scale.
How does this work for the error estimates? I then took their average and
computed the standard deviations of their differences from the average as a
function of time scale. That means taking the series at one year resolutions and
looking at the differences, 2 year averages, then the differences, 4 year, 10 year etc.
averaging over longer and longer periods and taking differences. The root mean
square of these differences is close to 0.05oC and – interestingly – it barely changes
with scale. If the corresponding variance is equally apportioned between the series,
this leads to about ±0.03 oC each. Since, we expect the overall error to decrease as
the square root of the averaging period, the near constancy of the error implies that
the errors and not independent of each other, this is an effect of the systematic
errors: because of this, the overall century scale errors are much larger (about ten
times larger), yet they are still very small!
References
Compo, G. P., P. D. Sardeshmukh, J. S. Whitaker, P. Brohan, P. D. Jones, and C. McColl
(2013), Independent confirmation of global land warming without the use of
station temperatures, Geophys. Res. Lett., 40, 3170–3174 doi: DOI:
10.1002/grl.50425. 9
Compo, G. P., et al. (2011), The Twentieth Century Reanalysis Project, Quarterly J.
Roy. Meteorol. Soc., 137, 1-28 doi: DOI: 10.1002/qj.776.
Lovejoy, S. (2013), What is climate?, EOS, 94, (1), 1 January, p1-2.
Lovejoy, S. (2014a), Scaling fluctuation analysis and statistical hypothesis testing of
anthropogenic warming, Climate Dynamics doi: 10.1007/s00382-014-2128-2.
Lovejoy, S. (2014b), A voyage through scales, a missing quadrillion and why the
climate is not what ou expect, Climate Dyn., (submitted, 2/14).
Lovejoy, S., and D. Schertzer (1986), Scale invariance in climatological temperatures
and the spectral plateau, Annales Geophysicae, 4B, 401-410.
Lovejoy, S., and D. Schertzer (2012a), Low frequency weather and the emergence of
the Climate, in Extreme Events and Natural Hazards: The Complexity
Perspective, edited by A. S. Sharma, A. Bunde, D. Baker and V. P. Dimri, pp. 231-
254, AGU monographs.
Lovejoy, S., and D. Schertzer (2012b), Stochastic and scaling climate sensitivities:
solar, volcanic and orbital forcings, Geophys. Res. Lett. , 39, L11702 doi:
doi:10.1029/2012GL051871.
Lovejoy, S., and D. Schertzer (2012c), Haar wavelets, fluctuations and structure
functions: convenient choices for geophysics, Nonlinear Proc. Geophys. , 19, 1-
14 doi: 10.5194/npg-19-1-2012.
Lovejoy, S., and D. Schertzer (2013), The Weather and Climate: Emergent Laws and
Multifractal Cascades, 496 pp., Cambridge University Press, Cambridge.
Lovejoy, S., D. Scherter, and D. Varon (2013), How scaling fluctuation analyses
change our view of the climate and its models (Reply to R. Pielke sr.:
Interactive comment on “Do GCM’s predict the climate… or macroweather?” by
S. Lovejoy et al.), Earth Syst. Dynam. Discuss., 3, C1–C12.
Mann, M. E., R. S. Bradley, and M. K. Hughes (1998), Global-scale temperature
patterns and climate forcing over the past six centuries, Nature, 392, 779-787.
For reasons beyond our control, and for an undetermined period of time, our comment section is now closed. However, our social networks remain open to your contributions.